本文為英文版的機器翻譯版本,如內容有任何歧義或不一致之處,概以英文版為準。
具名實體辨識
若要從非結構化文字擷取資訊並將其分類為預先定義的類別,請使用 Amazon G SageMaker round Truth 命名實體辨識 (NER) 標籤任務。傳統上,NER 包含篩選文字資料來尋找稱為「命名實體」的名詞片語,並使用標籤逐一進行分類,例如「人員」、「組織」或「品牌」。您可以將此任務擴大為標記較長的文字範圍,並使用您指定的預先定義標籤來分類那些文字序列。
在獲派具名實體辨識標記任務時,工作者會將您的標籤套用到較大文字區塊內的特定文字或片語。他們會選擇標籤,然後使用游標反白套用標籤的文字部分,以套用該標籤。Ground Truth 具名實體辨識工具支援重疊註釋、內容標籤選取,以及單一強調顯示多標籤選取。此外,工作者可以使用鍵盤快速選取標籤。
您可以使用 Amazon SageMaker 主控台的「Ground Truth」區段或作業建立具名實體辨識標籤任CreateLabelingJob
務。
重要
如果您手動建立輸入資訊清單檔案,請使用 "source"
來識別您要標記的文字。如需詳細資訊,請參閱 輸入資料。
建立具名實體辨識標記任務 (主控台)
您可以按照說明建立標記任務 (主控台)了解如何在 SageMaker 主控台中建立具名實體辨識標籤工作。在步驟 10 中,從任務類別下拉式清單中選擇文字,然後選擇具名實體辨識作為任務類型。
Ground Truth 提供類似下列標記任務的工作者 UI。使用主控台建立標記任務時,您可以指定指示以協助工作者完成任務,以及工作者可以選擇的標籤。
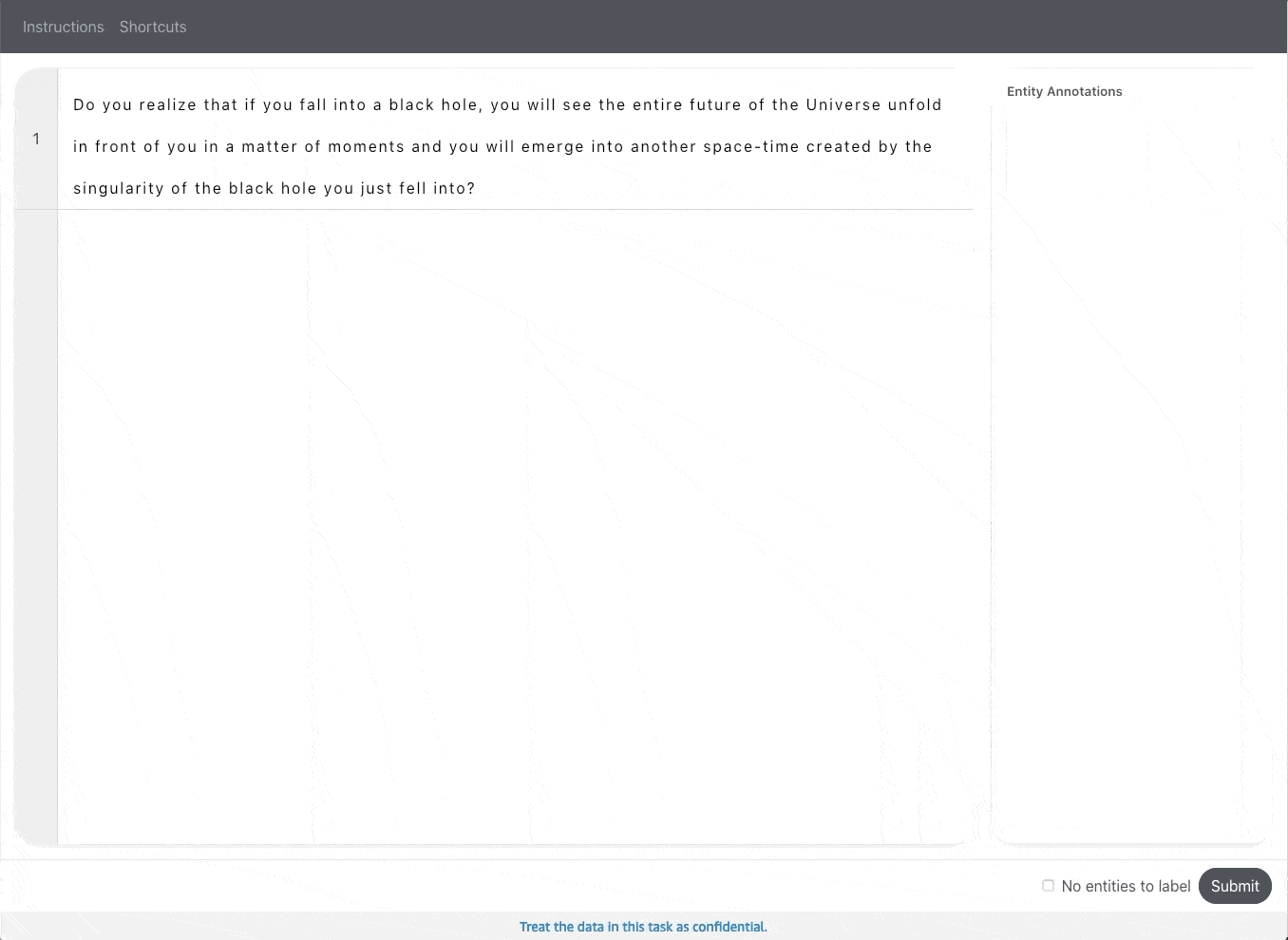
建立具名實體辨識標記任務 (API)
若要使用 SageMaker API 作業建立具名實體辨識標籤工作CreateLabelingJob
。此 API 為所有 AWS SDK 定義此操作。若要查看這項操作支援的特定語言 SDK 清單,請參閱 CreateLabelingJob
的〈另請參閱〉一節。
設定請求時,請遵循建立標記任務 (API) 上的指示並執行下列動作:
-
此任務類型的預先標註 Lambda 函數會以
PRE-NamedEntityRecognition
結尾。若要尋找您所在區域的預先註解 Lambda ARN,請參閱。PreHumanTaskLambdaArn -
此任務類型的註釋合併 Lambda 函數會以
ACS-NamedEntityRecognition
結尾。若要尋找適用於您區域的註解整合 Lambda ARN,請參閱。AnnotationConsolidationLambdaArn -
您必須為
HumanTaskUiArn
提供下列項目:arn:aws:sagemaker:
aws-region
:394669845002:human-task-ui/NamedEntityRecognition以您用來建立標記任務的 AWS 區域取代
。例如,在美國西部 (加利佛尼亞北部) 中建立標記任務時使用aws-region
us-west-1
。 -
使用
instructions
參數在標籤類別組態檔案中提供工作者指示。您可以在shortInstruction
和fullInstruction
欄位中使用字串或 HTML 標記語言。如需詳細資訊,請參閱在標籤類別組態檔案中提供工作者指示。"instructions": {"shortInstruction":"<h1>Add header</h1><p>Add Instructions</p>", "fullInstruction":"<p>Add additional instructions.</p>"}
下列是用 AWS Python 開發套件 (Boto3) 請求
response = client.create_labeling_job( LabelingJobName=
'example-ner-labeling-job'
, LabelAttributeName='label'
, InputConfig={ 'DataSource': { 'S3DataSource': { 'ManifestS3Uri':'s3://bucket/path/manifest-with-input-data.json'
} }, 'DataAttributes': { 'ContentClassifiers': ['FreeOfPersonallyIdentifiableInformation'|'FreeOfAdultContent'
, ] } }, OutputConfig={ 'S3OutputPath':'s3://bucket/path/file-to-store-output-data'
, 'KmsKeyId':'string'
}, RoleArn='arn:aws:iam::*:role/*'
, LabelCategoryConfigS3Uri='s3://bucket/path/label-categories.json'
, StoppingConditions={ 'MaxHumanLabeledObjectCount':123
, 'MaxPercentageOfInputDatasetLabeled':123
}, HumanTaskConfig={ 'WorkteamArn':'arn:aws:sagemaker:region:*:workteam/private-crowd/*'
, 'UiConfig': { 'HumanTaskUiArn': 'arn:aws:sagemaker:us-east-1
:394669845002:human-task-ui/NamedEntityRecognition' }, 'PreHumanTaskLambdaArn': 'arn:aws:lambda:us-east-1:432418664414
:function:PRE-NamedEntityRecognition', 'TaskKeywords': ['Named entity Recognition'
, ], 'TaskTitle':'Named entity Recognition task'
, 'TaskDescription':'Apply the labels provided to specific words or phrases within the larger text block.'
, 'NumberOfHumanWorkersPerDataObject':1
, 'TaskTimeLimitInSeconds':28800
, 'TaskAvailabilityLifetimeInSeconds':864000
, 'MaxConcurrentTaskCount':1000
, 'AnnotationConsolidationConfig': { 'AnnotationConsolidationLambdaArn': 'arn:aws:lambda:us-east-1:432418664414
:function:ACS-NamedEntityRecognition' }, Tags=[ { 'Key':'string'
, 'Value':'string'
}, ] )
在標籤類別組態檔案中提供工作者指示
您必須在使用 CreateLabelingJob
中的 LabelCategoryConfigS3Uri
參數識別的標籤類別組態檔案中提供工作者指示。您可以使用這些指示來提供您希望工作者執行的任務詳細資訊,並協助他們有效率地使用工具。
您可以分別使用 instructions
參數中的 shortInstruction
和 fullInstruction
提供短指示和長指示。若要深入了解這些指示類型,請參閱 建立說明頁面。
以下是標籤類別組態檔案的範例,其中包含可用於具名實體辨識標記任務的指示。
{ "document-version": "2018-11-28", "labels": [ { "label": "label1", "shortDisplayName": "L1" }, { "label": "label2", "shortDisplayName": "L2" }, { "label": "label3", "shortDisplayName": "L3" }, { "label": "label4", "shortDisplayName": "L4" }, { "label": "label5", "shortDisplayName": "L5" } ], "instructions": { "shortInstruction": "<p>Enter description of the labels that workers have to choose from</p><br><p>Add examples to help workers understand the label</p>", "fullInstruction": "<ol> <li><strong>Read</strong> the text carefully.</li> <li><strong>Highlight</strong> words, phrases, or sections of the text.</li> <li><strong>Choose</strong> the label that best matches what you have highlighted.</li> <li>To <strong>change</strong> a label, choose highlighted text and select a new label.</li> <li>To <strong>remove</strong> a label from highlighted text, choose the X next to the abbreviated label name on the highlighted text.</li> <li>You can select all of a previously highlighted text, but not a portion of it.</li> </ol>" } }
具名實體辨識輸出資料
建立具名實體辨識標記任務後,您的輸出資料會位於使用 API 時在 S3OutputPath
參數中指定的 Amazon S3 儲存貯體中,或在主控台標記任務區段的輸出資料集位置中。
若要進一步了解 Ground Truth 產生的輸出資訊清單檔案,以及 Ground Truth 用來儲存輸出資料的檔案結構,請參閱 輸出資料。