Medical imaging
Medical imaging in healthcare spans diagnostic medical imaging,
digital pathology, dental imaging, and related applications.
Medical imaging systems enable workflows anchored in the
generation, storage, and analysis of medical imaging study data.
The data is commonly generated by medical imaging hardware, like
CT
scanner
Characteristics of medical imaging architectures include:
-
Imaging study data is generated by on-premises modalities, durably stored by PACS and VNA solutions, and maintained highly available for immediate retrieval by radiologists and other end users. Solution end users, like radiologists, likely expect low latency retrievals of new and old imaging studies.
-
An imaging study will likely be accessed several times shortly after ingestion, and tends to be accessed less frequently with age. Regulatory requirements may dictate that studies be retained for several years, and operators may decide to keep studies in perpetuity.
-
The imaging scanners, physicians, technicians, and radiologists may be located at the same physical site. Or, cross-site collaboration and outsourced radiology services may extend workflows across multiple care settings.
-
Medical imaging systems commonly interoperate with other provider systems, like EHRs, though data integrations.
-
Medical imaging study data is often stored and transmitted using the DICOM standard
. This standard covers both a network protocol and file format definitions. -
Some medical imaging solutions, such PACS, may fall under regulatory control, such as GxP regulation
in the US. Machine learning algorithms that aid in diagnoses may also fall under regulatory control. Determine which, if any, regulatory controls are applicable to your solutions. -
Data processing, provider workflows, and end user interfaces may leverage AI to improve care quality and boost productivity. Example applications include computer vision applied to detect disease in medical images, and NLP applied to support report authoring.
-
A high-level overview of the medical imaging workflow is shown in figure 3.
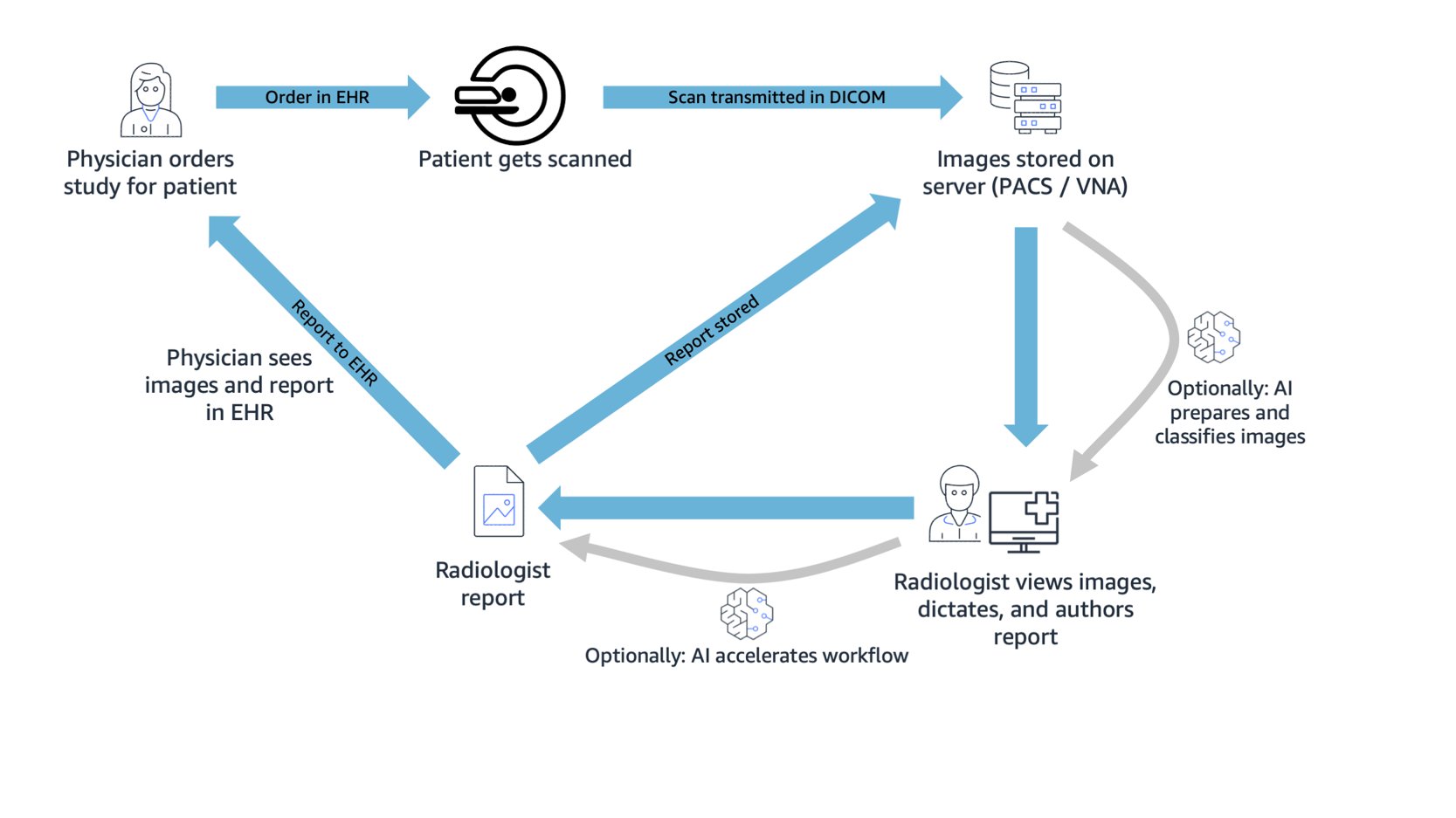
A representative medical imaging workflow.