Lambda integrates with AWS X-Ray to help you trace, debug, and optimize Lambda applications. You can use X-Ray to trace a request as it traverses resources in your application, which may include Lambda functions and other AWS services.
To send tracing data to X-Ray, you can use one of three SDK libraries:
-
AWS Distro for OpenTelemetry (ADOT)
– A secure, production-ready, AWS-supported distribution of the OpenTelemetry (OTel) SDK. -
AWS X-Ray SDK for Python – An SDK for generating and sending trace data to X-Ray.
-
Powertools for AWS Lambda (Python)
– A developer toolkit to implement Serverless best practices and increase developer velocity.
Each of the SDKs offer ways to send your telemetry data to the X-Ray service. You can then use X-Ray to view, filter, and gain insights into your application's performance metrics to identify issues and opportunities for optimization.
Important
The X-Ray and Powertools for AWS Lambda SDKs are part of a tightly integrated instrumentation solution offered by AWS. The ADOT Lambda Layers are part of an industry-wide standard for tracing instrumentation that collect more data in general, but may not be suited for all use cases. You can implement end-to-end tracing in X-Ray using either solution. To learn more about choosing between them, see Choosing between the AWS Distro for Open Telemetry and X-Ray SDKs.
Sections
Using Powertools for AWS Lambda (Python) and AWS SAM for tracing
Follow the steps below to download, build, and deploy a sample Hello World Python application with integrated Powertools for AWS Lambda (Python)
Prerequisites
To complete the steps in this section, you must have the following:
-
Python 3.11
-
AWS SAM CLI version 1.75 or later. If you have an older version of the AWS SAM CLI, see Upgrading the AWS SAM CLI.
Deploy a sample AWS SAM application
-
Initialize the application using the Hello World Python template.
sam init --app-template hello-world-powertools-python --name sam-app --package-type Zip --runtime python3.11 --no-tracing
-
Build the app.
cd sam-app && sam build
-
Deploy the app.
sam deploy --guided
-
Follow the on-screen prompts. To accept the default options provided in the interactive experience, press
Enter
.Note
For HelloWorldFunction may not have authorization defined, Is this okay?, make sure to enter
y
. -
Get the URL of the deployed application:
aws cloudformation describe-stacks --stack-name sam-app --query 'Stacks[0].Outputs[?OutputKey==`HelloWorldApi`].OutputValue' --output text
-
Invoke the API endpoint:
curl -X GET
<URL_FROM_PREVIOUS_STEP>
If successful, you'll see this response:
{"message":"hello world"}
-
To get the traces for the function, run sam traces.
sam traces
The trace output looks like this:
New XRay Service Graph Start time: 2023-02-03 14:59:50+00:00 End time: 2023-02-03 14:59:50+00:00 Reference Id: 0 - (Root) AWS::Lambda - sam-app-HelloWorldFunction-YBg8yfYtOc9j - Edges: [1] Summary_statistics: - total requests: 1 - ok count(2XX): 1 - error count(4XX): 0 - fault count(5XX): 0 - total response time: 0.924 Reference Id: 1 - AWS::Lambda::Function - sam-app-HelloWorldFunction-YBg8yfYtOc9j - Edges: [] Summary_statistics: - total requests: 1 - ok count(2XX): 1 - error count(4XX): 0 - fault count(5XX): 0 - total response time: 0.016 Reference Id: 2 - client - sam-app-HelloWorldFunction-YBg8yfYtOc9j - Edges: [0] Summary_statistics: - total requests: 0 - ok count(2XX): 0 - error count(4XX): 0 - fault count(5XX): 0 - total response time: 0 XRay Event [revision 1] at (2023-02-03T14:59:50.204000) with id (1-63dd2166-434a12c22e1307ff2114f299) and duration (0.924s) - 0.924s - sam-app-HelloWorldFunction-YBg8yfYtOc9j [HTTP: 200] - 0.016s - sam-app-HelloWorldFunction-YBg8yfYtOc9j - 0.739s - Initialization - 0.016s - Invocation - 0.013s - ## lambda_handler - 0.000s - ## app.hello - 0.000s - Overhead
-
This is a public API endpoint that is accessible over the internet. We recommend that you delete the endpoint after testing.
sam delete
X-Ray doesn't trace all requests to your application. X-Ray applies a sampling algorithm to ensure that tracing is efficient, while still providing a representative sample of all requests. The sampling rate is 1 request per second and 5 percent of additional requests. You can't configure the X-Ray sampling rate for your functions.
Using Powertools for AWS Lambda (Python) and the AWS CDK for tracing
Follow the steps below to download, build, and deploy a sample Hello World Python application with integrated Powertools for AWS Lambda (Python)
Prerequisites
To complete the steps in this section, you must have the following:
-
Python 3.11
-
AWS SAM CLI version 1.75 or later. If you have an older version of the AWS SAM CLI, see Upgrading the AWS SAM CLI.
Deploy a sample AWS CDK application
-
Create a project directory for your new application.
mkdir hello-world cd hello-world
-
Initialize the app.
cdk init app --language python
-
Install the Python dependencies.
pip install -r requirements.txt
Create a directory lambda_function under the root folder.
mkdir lambda_function cd lambda_function
Create a file app.py and add the following code to the file. This is the code for the Lambda function.
from aws_lambda_powertools.event_handler import APIGatewayRestResolver from aws_lambda_powertools.utilities.typing import LambdaContext from aws_lambda_powertools.logging import correlation_paths from aws_lambda_powertools import Logger from aws_lambda_powertools import Tracer from aws_lambda_powertools import Metrics from aws_lambda_powertools.metrics import MetricUnit app = APIGatewayRestResolver() tracer = Tracer() logger = Logger() metrics = Metrics(namespace="PowertoolsSample") @app.get("/hello") @tracer.capture_method def hello(): # adding custom metrics # See: https://docs.powertools.aws.dev/lambda-python/latest/core/metrics/ metrics.add_metric(name="HelloWorldInvocations", unit=MetricUnit.Count, value=1) # structured log # See: https://docs.powertools.aws.dev/lambda-python/latest/core/logger/ logger.info("Hello world API - HTTP 200") return {"message": "hello world"} # Enrich logging with contextual information from Lambda @logger.inject_lambda_context(correlation_id_path=correlation_paths.API_GATEWAY_REST) # Adding tracer # See: https://docs.powertools.aws.dev/lambda-python/latest/core/tracer/ @tracer.capture_lambda_handler # ensures metrics are flushed upon request completion/failure and capturing ColdStart metric @metrics.log_metrics(capture_cold_start_metric=True) def lambda_handler(event: dict, context: LambdaContext) -> dict: return app.resolve(event, context)
-
Open the hello_world directory. You should see a file called hello_world_stack.py.
cd .. cd hello_world
Open hello_world_stack.py and add the following code to the file. This contains the Lambda Constructor, which creates the Lambda function, configures environment variables for Powertools and sets log retention to one week, and the ApiGatewayv1 Constructor, which creates the REST API.
from aws_cdk import ( Stack, aws_apigateway as apigwv1, aws_lambda as lambda_, CfnOutput, Duration ) from constructs import Construct class HelloWorldStack(Stack): def __init__(self, scope: Construct, construct_id: str, **kwargs) -> None: super().__init__(scope, construct_id, **kwargs) # Powertools Lambda Layer powertools_layer = lambda_.LayerVersion.from_layer_version_arn( self, id="lambda-powertools", # At the moment we wrote this example, the aws_lambda_python_alpha CDK constructor is in Alpha, o we use layer to make the example simpler # See https://docs.aws.amazon.com/cdk/api/v2/python/aws_cdk.aws_lambda_python_alpha/README.html # Check all Powertools layers versions here: https://docs.powertools.aws.dev/lambda-python/latest/#lambda-layer layer_version_arn=f"arn:aws:lambda:{self.region}:017000801446:layer:AWSLambdaPowertoolsPythonV2:21" ) function = lambda_.Function(self, 'sample-app-lambda', runtime=lambda_.Runtime.PYTHON_3_11, layers=[powertools_layer], code = lambda_.Code.from_asset("./lambda_function/"), handler="app.lambda_handler", memory_size=128, timeout=Duration.seconds(3), architecture=lambda_.Architecture.X86_64, environment={ "POWERTOOLS_SERVICE_NAME": "PowertoolsHelloWorld", "POWERTOOLS_METRICS_NAMESPACE": "PowertoolsSample", "LOG_LEVEL": "INFO" } ) apigw = apigwv1.RestApi(self, "PowertoolsAPI", deploy_options=apigwv1.StageOptions(stage_name="dev")) hello_api = apigw.root.add_resource("hello") hello_api.add_method("GET", apigwv1.LambdaIntegration(function, proxy=True)) CfnOutput(self, "apiUrl", value=f"{apigw.url}hello")
-
Deploy your application.
cd .. cdk deploy
-
Get the URL of the deployed application:
aws cloudformation describe-stacks --stack-name HelloWorldStack --query 'Stacks[0].Outputs[?OutputKey==`apiUrl`].OutputValue' --output text
-
Invoke the API endpoint:
curl -X GET
<URL_FROM_PREVIOUS_STEP>
If successful, you'll see this response:
{"message":"hello world"}
-
To get the traces for the function, run sam traces.
sam traces
The traces output looks like this:
New XRay Service Graph Start time: 2023-02-03 14:59:50+00:00 End time: 2023-02-03 14:59:50+00:00 Reference Id: 0 - (Root) AWS::Lambda - sam-app-HelloWorldFunction-YBg8yfYtOc9j - Edges: [1] Summary_statistics: - total requests: 1 - ok count(2XX): 1 - error count(4XX): 0 - fault count(5XX): 0 - total response time: 0.924 Reference Id: 1 - AWS::Lambda::Function - sam-app-HelloWorldFunction-YBg8yfYtOc9j - Edges: [] Summary_statistics: - total requests: 1 - ok count(2XX): 1 - error count(4XX): 0 - fault count(5XX): 0 - total response time: 0.016 Reference Id: 2 - client - sam-app-HelloWorldFunction-YBg8yfYtOc9j - Edges: [0] Summary_statistics: - total requests: 0 - ok count(2XX): 0 - error count(4XX): 0 - fault count(5XX): 0 - total response time: 0 XRay Event [revision 1] at (2023-02-03T14:59:50.204000) with id (1-63dd2166-434a12c22e1307ff2114f299) and duration (0.924s) - 0.924s - sam-app-HelloWorldFunction-YBg8yfYtOc9j [HTTP: 200] - 0.016s - sam-app-HelloWorldFunction-YBg8yfYtOc9j - 0.739s - Initialization - 0.016s - Invocation - 0.013s - ## lambda_handler - 0.000s - ## app.hello - 0.000s - Overhead
-
This is a public API endpoint that is accessible over the internet. We recommend that you delete the endpoint after testing.
cdk destroy
Using ADOT to instrument your Python functions
ADOT provides fully managed Lambda layers that package
everything you need to collect telemetry data using the OTel SDK. By consuming this layer, you can instrument your
Lambda functions without having to modify any function code. You can also configure your layer to do custom
initialization of OTel. For more information, see Custom configuration for the ADOT Collector on Lambda
For Python runtimes, you can add the AWS managed Lambda layer for ADOT Python
to automatically instrument your functions. This layer works for both arm64 and x86_64 architectures. For detailed
instructions on how to add this layer, see AWS Distro for
OpenTelemetry Lambda Support for Python
Using the X-Ray SDK to instrument your Python functions
To record details about calls that your Lambda function makes to other resources in your application, you can
also use the AWS X-Ray SDK for Python. To get the SDK, add the aws-xray-sdk
package to your application's
dependencies.
Example requirements.txt
jsonpickle==1.3 aws-xray-sdk==2.4.3
In your function code, you can instrument AWS SDK clients by patching the boto3
library
with the aws_xray_sdk.core
module.
Example function – Tracing
an AWS SDK client
import boto3 from aws_xray_sdk.core import xray_recorder from aws_xray_sdk.core import patch_all logger = logging.getLogger() logger.setLevel(logging.INFO) patch_all() client = boto3.client('lambda') client.get_account_settings() def lambda_handler(event, context): logger.info('## ENVIRONMENT VARIABLES\r' + jsonpickle.encode(dict(**os.environ))) ...
After you add the correct dependencies and make the necessary code changes, activate tracing in your function's configuration via the Lambda console or the API.
Activating tracing with the Lambda console
To toggle active tracing on your Lambda function with the console, follow these steps:
To turn on active tracing
Open the Functions page
of the Lambda console. -
Choose a function.
Choose Configuration and then choose Monitoring and operations tools.
Under Additional monitoring tools, choose Edit.
-
Under CloudWatch Application Signals and AWS X-Ray, choose Enable for Lambda service traces.
-
Choose Save.
Activating tracing with the Lambda API
Configure tracing on your Lambda function with the AWS CLI or AWS SDK, use the following API operations:
The following example AWS CLI command enables active tracing on a function named my-function.
aws lambda update-function-configuration --function-name my-function \ --tracing-config Mode=Active
Tracing mode is part of the version-specific configuration when you publish a version of your function. You can't change the tracing mode on a published version.
Activating tracing with AWS CloudFormation
To activate tracing on an AWS::Lambda::Function
resource in an AWS CloudFormation template, use the
TracingConfig
property.
Example function-inline.yml –
Tracing configuration
Resources: function: Type: AWS::Lambda::Function Properties:
TracingConfig: Mode: Active
...
For an AWS Serverless Application Model (AWS SAM) AWS::Serverless::Function
resource, use the Tracing
property.
Example template.yml – Tracing
configuration
Resources: function: Type: AWS::Serverless::Function Properties:
Tracing: Active
...
Interpreting an X-Ray trace
Your function needs permission to upload trace data to X-Ray. When you activate tracing in the Lambda
console, Lambda adds the required permissions to your function's execution role. Otherwise, add the AWSXRayDaemonWriteAccess
After you've configured active tracing, you can observe specific requests through your application. The X-Ray service graph shows information about your application and all its components. The following example shows an application with two functions. The primary function processes events and sometimes returns errors. The second function at the top processes errors that appear in the first's log group and uses the AWS SDK to call X-Ray, Amazon Simple Storage Service (Amazon S3), and Amazon CloudWatch Logs.

X-Ray doesn't trace all requests to your application. X-Ray applies a sampling algorithm to ensure that tracing is efficient, while still providing a representative sample of all requests. The sampling rate is 1 request per second and 5 percent of additional requests. You can't configure the X-Ray sampling rate for your functions.
In X-Ray, a trace records information about a request that is processed by one or more services. Lambda records 2 segments per trace, which creates two nodes on the service graph. The following image highlights these two nodes:
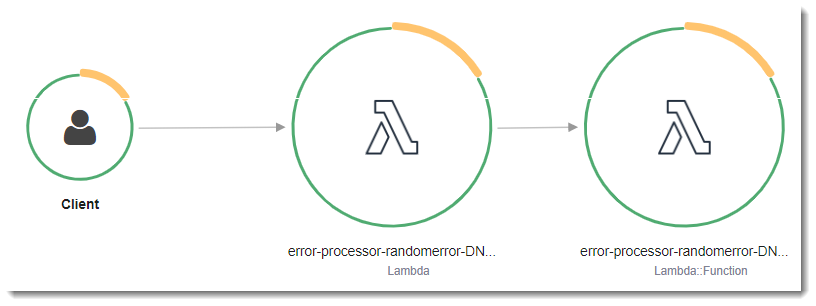
The first node on the left represents the Lambda service, which receives the invocation request. The second
node represents your specific Lambda function. The following example shows a trace with these two segments. Both
are named my-function, but one has an origin of AWS::Lambda
and the other has
an origin of AWS::Lambda::Function
. If the AWS::Lambda
segment shows an error, the Lambda service had an issue. If the AWS::Lambda::Function
segment shows an error, your function had an issue.

This example expands the AWS::Lambda::Function
segment to show its three subsegments.
Note
AWS is currently implementing changes to the Lambda service. Due to these changes, you may see minor differences between the structure and content of system log messages and trace segments emitted by different Lambda functions in your AWS account.
The example trace shown here illustrates the old-style function segment. The differences between the old- and new-style segments are described in the following paragraphs.
These changes will be implemented during the coming weeks, and all functions in all AWS Regions except the China and GovCloud regions will transition to use the new-format log messages and trace segments.
The old-style function segment contains the following subsegments:
-
Initialization – Represents time spent loading your function and running initialization code. This subsegment only appears for the first event that each instance of your function processes.
-
Invocation – Represents the time spent running your handler code.
-
Overhead – Represents the time the Lambda runtime spends preparing to handle the next event.
The new-style function segment doesn't contain an Invocation
subsegment. Instead,
customer subsegments are attached directly to the function segment. For more information about the structure of the
old- and new-style function segments, see Understanding X-Ray traces.
You can also instrument HTTP clients, record SQL queries, and create custom subsegments with annotations and metadata. For more information, see the AWS X-Ray SDK for Python in the AWS X-Ray Developer Guide.
Pricing
You can use X-Ray tracing for free each month up to a certain limit as part of the AWS Free Tier. Beyond that threshold, X-Ray charges for trace storage and
retrieval. For more information, see AWS X-Ray pricing
Storing runtime dependencies in a layer (X-Ray SDK)
If you use the X-Ray SDK to instrument AWS SDK clients your function code, your deployment package can become quite large. To avoid uploading runtime dependencies every time you update your function code, package the X-Ray SDK in a Lambda layer.
The following example shows an AWS::Serverless::LayerVersion
resource that stores the
AWS X-Ray SDK for Python.
Example template.yml – Dependencies
layer
Resources: function: Type: AWS::Serverless::Function Properties: CodeUri: function/. Tracing: Active
Layers: - !Ref libs
...libs: Type: AWS::Serverless::LayerVersion Properties: LayerName: blank-python-lib Description: Dependencies for the blank-python sample app. ContentUri: package/. CompatibleRuntimes: - python3.11
With this configuration, you update the library layer only if you change your runtime dependencies. Since the function deployment package contains only your code, this can help reduce upload times.
Creating a layer for dependencies requires build changes to generate the layer archive prior to deployment.
For a working example, see the blank-python